AI Powered Weapon Detection System.
Transforming perimeter screening & security – replacing obsolete walkthrough metal detectors.
Effortlessly detect weapons and security threats, while enhancing experience.
Xtract One’s integrated security solutions not only secure venues, they secure great times. Treat your patrons like the valued guests they are by providing a frictionless welcoming experience from the moment they arrive at your venue. With Xtract One’s integrated security solutions, metal detectors, long lines, delays and invasive pat downs become obsolete.
Xtract One’s touchless, non-invasive, integrated threat detection applications such as gateway patron screening, video recognition and real-time analytics capabilities remove the need for manual security monitoring as well as the invasion of your patron’s privacy.
Technology that helps security and venue operations work smarter.
Xtract One is the industry-disrupting, AI-powered security technology company propelling the physical security industry into the digital-first world. Xtract One’s software-based security solutions include a range of integrated applications designed to preserve patron experience, streamline operations and provide actionable insights, transforming the industry from a reactive to proactive model. Personnel are alerted to potential threats instantly, gaining the ability to make faster, informed and proactive decisions.
You only get one chance to make a first impression.
Make it memorable.
Attending a high-volume event in a large venue can cause patrons to go from feeling excited to overwhelmed in an instant. With Xtract One’s touchless, frictionless security solutions, your patrons are made to feel welcome, and they will remember. Good memories are priceless, and great memories are built on seamless experiences.
The Xtract One
Advantage
For Venue Operators
Shift your security operations from reactive to proactive
Enhance safety of your patrons without sacrificing experience
Reduce unnecessary labor so personnel can reallocate time where most effective
Optimize patron experience to encourage loyalty and conversions
For Patrons
Welcoming, frictionless experience
Comfort without sacrificing safety
Eliminated screening bias or discrimination
Safety and security without invading privacy
Products

SafeGateway is an accurate, fast, and unobtrusive solution to seamlessly secure patron and employee entrances. This innovative solution automatically scans entrants for concealed weapons such as guns, knives, and IED components more efficiently and accurately than conventional security measures like metal detectors. This technology reduces or eliminates long lineups, uncomfortable pat downs and invasive searches. SafeGateway is the ideal choice for stadiums, arenas, live entertainment venues, theaters, casinos, nightclubs, art galleries, and other attractions that require effective security screening, without detracting from the guest experience.
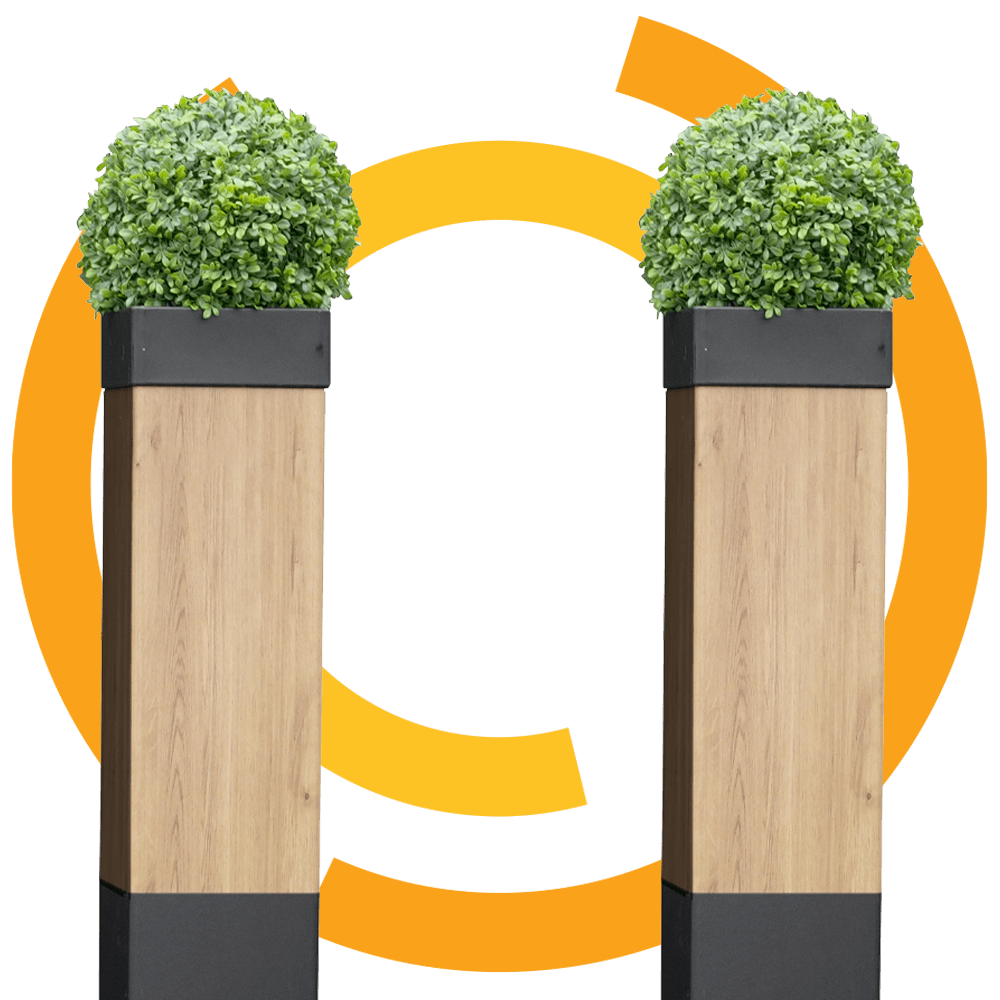

SmartGateway delivers fast, reliable, and accurate patron screening for high through-put venues, replacing intimidating metal detectors.This solution unobtrusively scans patrons for guns, knives, and other prohibited items as they enter your facility, using AI-powered sensors to detect threats without invading the sense of privacy and comfort of your patrons. This system is ideal for stadiums and other ticketed venues that need to get thousands of people in quickly, safely, and in alignment with security standards requirements.
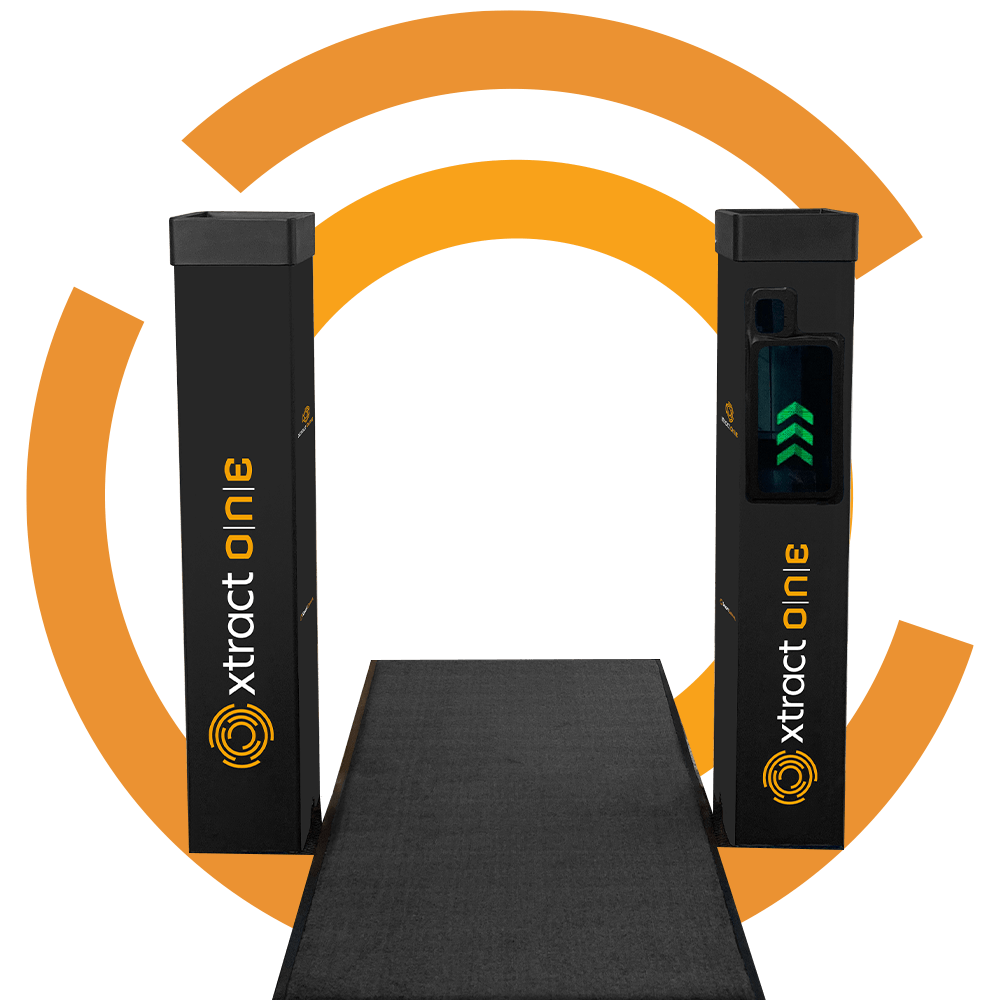
An intuitive, single-window overview of your entire operation.
Change settings in a moment, from anywhere. Access data, from anywhere. Check system health, from anywhere. For all of your Gateways, in one place.
Xtract One’s cloud-based platform, Xtract One View, provides oversight of your entire fleet of Xtract One SmartGateways from one interface. Different entrances, different facilities, even different continents – wherever your SmartGateways are, Xtract One View provides a central management system from which to monitor and manage all your SmartGateways and analyze your facility ingress.
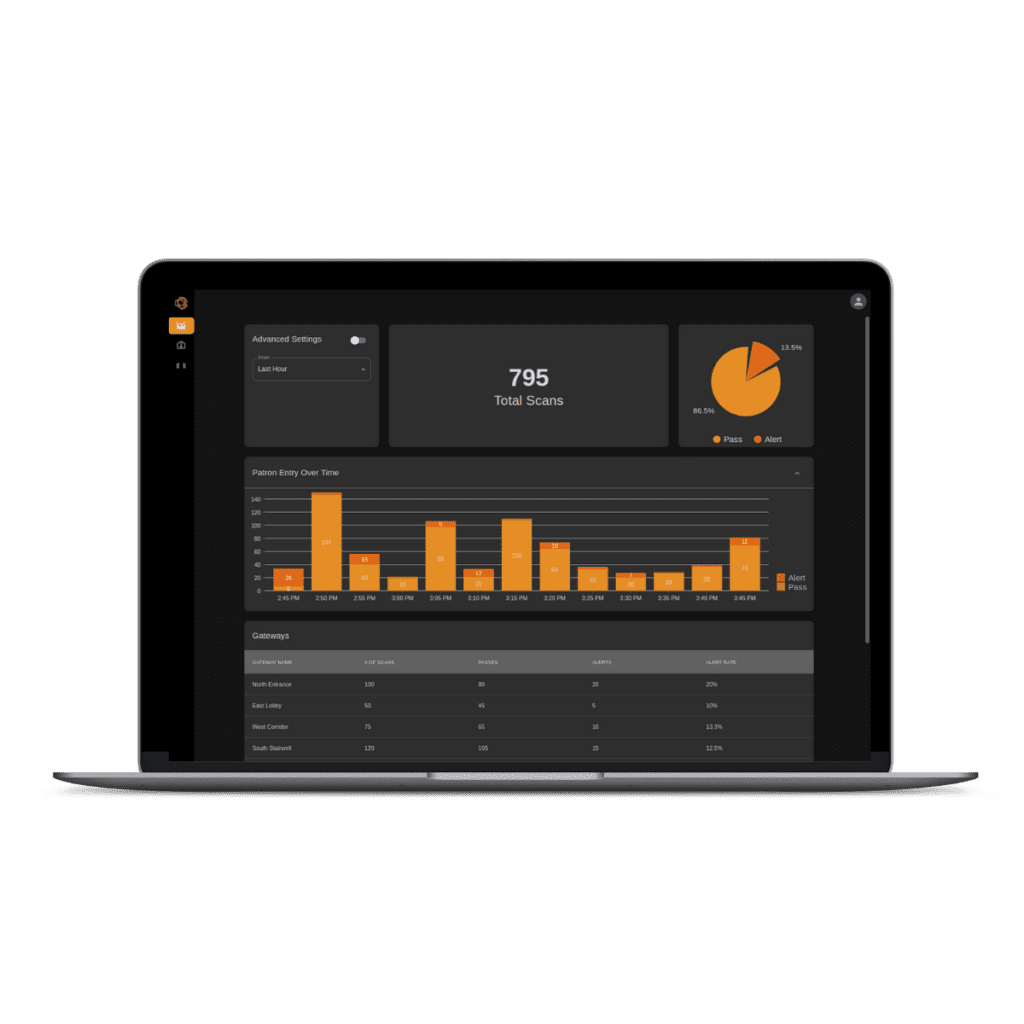
Hear from our Clients
Latest News
Xtract One Closes $7.2M Public Offering and $1.4M Investment by Strategic Partner
THIS NEWS RELEASE IS INTENDED FOR DISTRIBUTION IN CANADA ONLY AND IS NOT INTENDED FOR DISTRIBUTION TO UNITED STATES NEWSWIRE
2024-04-24The Stadium Business Summit & Venue Technology Showcase
Xtract One Technologies will be attending The Stadium Business Summit & Venue Technology Showcase on June 18 – June 19,
2024-04-23RTM Spring School Facility and Safety Congress
Xtract One Technologies will be attending the RTM Spring School Facility and Safety Congress on May 5- May 7, 2024, at
2024-04-23